The greatest bubbles need a transformational technology and a ripe macro and monetary backdrop, and we now have both.
I don't generally write reports in the first person, because most everything I do here at Bridgewater is part of a culture of compounded understanding that is generated by a process that is much bigger than me. This wire, too, benefits from great contributions by many of my colleagues, but the likely errors and wild guesses in it are uniquely my own, so I don’t want them to be misattributed to my more wise and cautious colleagues. Luckily, my co-authors here, Atul and Alex, are willing to go along for the ride.
I believe the AI bubble is ahead of us, not behind us. The greatest bubbles need a truly transformational technology and a ripe macro and monetary environment. We now have both.
The recent disappointment around Nvidia’s quarterly earnings and the market action since then now offers a good opportunity to share my thoughts on how much of the AI story is still to come and to address some of the doubts creeping into the market.
The doubts I am hearing include:
- It’s all priced in. Nvidia’s earnings were great by any reasonable measure and beat the analyst estimates on record, but that was not enough to get a rally on the news, indicating the stock/theme is fully priced.
- Where’s the revenue? LLMs (large language models) aren’t translating into significant revenue yet, let alone profits, sowing doubt that they will over any reasonable time frame.
- LLMs are dumb anyway. Many users have trouble getting LLMs to produce consistently good answers, particularly on multistep problems, and that has soured some on their promise.
- LLM progress is slowing. New models are only outperforming older ones by modest amounts, but it costs more and more to make those marginal improvements.
- Been there, done that. Some seasoned investors argue that AI optimism comes along every decade or so and always fizzles out, and suspect this is another one of those.
I disagree with most of the above and will describe why below, but to be clear, there are big things I worry about:
- In its current form, AI is easier to use for destructive purposes than productive ones. It is possible that a very public, very negative use case for AI will impact the landscape before productive ones do. In many senses, this is already the case, as the application of narrow AI to content algorithms has likely contributed to the negative impacts of social media on society. I don’t think it will be long before it becomes obvious that AI is being used to commit crimes.
- Regulation: if AI is as impactful as I expect, serious regulation will take place, probably following a major disaster. The scope of regulation is huge, as there are so many issues (impact on the labor market, copyrights, privacy, etc.) and will likely have material impact on how much of the benefit is harvested.
- It’s hard to say who the winners will be and if they will hold on to their winnings. Will the rents go to the people who develop the technology, to those who deploy it well, to consumer surplus, or to something else? Will the first movers maintain moats or be crushed by later movers who can deliver the technology at a lower price? What types of chips will be used in the future? What types of energy will end up being needed?
All things considered, I net to: we ain’t seen nothing yet. I say that having struggled with the promises and challenges of machine intelligence for decades—first through my part in building out one of the most comprehensive expert systems, then over the past 10 years through being involved with the machine learning community. Since then, I have been thinking about and working on the ingredients I considered necessary for machine intelligence to compete with human intelligence on most tasks. By the end of 2022, I believed that the technology was finally poised to get there over the next 5-7 years and so chose that moment to dive in. I founded AIA Labs at Bridgewater at the beginning of 2023 with the mission to build a wholly artificial investor that can outcompete humans. We will see, but that is our intent. Everything I have seen since then has made me believe that thesis more, not less. And that is despite the fact that LLMs have turned out so far to be almost comically bad planners—an important facet of human intelligence. Yes, machine intelligence is frustrating and large leaps still need to be made to supplant humans in many fields, but to my eye, we are at the precipice.
What I Am Looking For Over the Next Few Years
Among other things, a much broader investment boom. So far, investment in AI has mostly been narrow; massive dollar amounts have been spent but by relatively few players. Some market watchers see this as a risk because the revenues from building out that capacity are not diversified (largely going from one firm in this tight circle to another). I disagree with this interpretation. What I see is that the people who know the most are spending a lot and the pace of their spending is accelerating, while so many others are not investing at all. I think spending by the major tech names (and some small startups) will continue to accelerate.
One way this could play out is that, eventually, one of those startups with very few employees and many AI agents crushes traditional rivals—in a sector outside of traditional tech. That then sets off panic capex in nearly all sectors as a risk that used to be existential to the Googles of the world becomes existential across the economy. If I had to guess, I’d say we are about 18 months from that realization, but I don’t want to suggest any false precision here.
AI Progress Has Occurred at a Faster and Faster Pace, Yielding Very Impressive Raw Capabilities
Where AI could go from here (and how quickly) necessarily starts with a) what it can do today and b) how fast it is advancing. The answer is a) a lot and b) fast—and getting faster. The chart below maps out the time elapsed before AI reached (and ultimately exceeded) benchmarks of human-level performance across a range of capabilities. From the start of this century, it took 12 years to reach human-level performance in handwriting recognition. Since then, AI systems have demonstrated human-level capabilities in progressively more challenging arenas, first tackling speech and image recognition, then visual reasoning, then language reasoning. But, as you can see on the chart, even as the benchmarks got more difficult, it took less time for AI to reach proficiency. In other words, when you consider the increase in difficulty, the pace of progress accelerated even more dramatically than this two-dimensional chart can convey.
Finally, the red line on the far right of the chart shows AI systems reaching human-level proficiency in competitive math, which is about as different from language as one can get. In 2021, AI systems were only correctly answering about 6-7% of competition-level math questions and failed to consistently grasp basic relationships; a common geometry mistake involved calculating the area of a rectangle by adding the length and width together instead of multiplying them. But after just three years, their accuracy improved to around 90%. As I discuss below, that leap was made by combining LLMs with symbolic reasoning engines, an approach that has the potential to make further huge leaps from here.

Obstacles on the Road to Agentic AI—and the Path Beyond
At this point, the raw power is clear. The next problem to crack is how to harness those strengths into usable agents. My colleagues at AIA Labs and I are looking to see how much progress AI agents can make on becoming better planners and error correctors, as well as how easy it could become to plug them into other applications that confirm their work.
There are key limitations to continued progress along this path that I want to lay out clearly before addressing.
- First, compute and data are not infinite, and all else equal, this imposes a very real limit. Advances in LLMs have so far been enabled by the rapid increase in computing power and the availability of vast amounts of data. However, it is not a foregone conclusion that increasing resources will continue to produce performance improvements: we are rapidly exhausting the supply of existing high-quality text data available to train LLMs, and it remains an open question whether this “data wall” will be overcome. If current trends continue, language models will fully utilize the stock of public human-generated text data before the end of this decade, possibly as early as 2026. It’s not clear how much additive value can be squeezed from synthetic data, which is itself often AI-generated, or from tapping into vast amounts of visual data.
- Beyond this, LLMs (currently) have crippling functional shortcomings that limit their broader application. In addition to the problem of hallucination, current-generation LLMs struggle to plan more than a few steps ahead. Without greater planning abilities, it will likely remain difficult for LLMs to independently perform complex tasks. It remains to be seen whether scale alone will enable LLMs to overcome this limitation.
I believe (and this view is shared by our AIA Labs Chief Scientist Jas Sekhon) that many of the current limitations of LLMs will be overcome over the next three or so years. And even if some remain unresolved, the technology can still be transformative for productivity.
We see two potential paths to widespread impact:
- A future where LLMs can plan and act with substantially more autonomy and capability. If generative AI systems can overcome their limitations with simply more compute and more data, then the recent progress that AI has made would look slow. If they become smarter and develop better planning capabilities, LLMs could evolve into independent workers that could be dropped into existing organizations with limited setup costs. They could transition from being, for example, programming assistants for developers to independently designing and implementing large-scale software projects. In this world, the key consideration would be how best to integrate your new AI coworkers into your existing data and systems. And in a world where we do get to Artificial General Intelligence (AGI)—a hypothetical form of AI that would possess the ability to understand, learn, and apply intelligence across a wide range of tasks at a level equal to or surpassing human cognitive abilities—the resulting innovation would be unprecedented in human history, on par with the Industrial Revolution.
A future where LLMs continue to improve but remain limited to relatively narrow tasks. The other possibility is that LLMs do not improve much at planning and retain many of their current limitations, even as they scale. This does not mean that applications for LLMs will remain as limited as they are today, but it does mean that it will take more work to develop them and that these applications will likely take a different form.
A reason for optimism even if we follow this path is that, despite their current limitations, progress has been rapid on challenging scientific tasks when LLMs have been combined with other AI and ML tools that mitigate their weakness. While recent progress with LLMs has been impressive and headline-grabbing, generative AI is just one application of machine learning. Machine learning more broadly has seen tremendous advances in recent years and is powering major scientific developments and large swaths of economic activity, from robotics and genomics to search, advertising, content delivery, and market design applications used by leading tech firms. Even if progress with generative AI slows or stalls, progress in these other areas will likely continue and new applications for machine learning will likely be found.
Even with their shortcomings today, LLMs are already proving to be extremely powerful when paired with other tools. For example, while off-the-shelf LLMs struggle with elementary arithmetic, Google DeepMind’s AlphaGeometry system—which integrates an LLM with a symbolic reasoning engine—can solve extremely challenging geometry problems at a rate which rivals that of the world’s best mathematicians.
Either scenario could unleash productivity and impact at a scale I do not believe markets are pricing in today. Add that to the Fed proactively easing to support the labor market, still-solid growth, and healthy private sector balance sheets, and you have the ingredients for a further equity rally, with the possibility that rational exuberance about a transformative technology becomes irrational, fueling a bubble.
In the rest of this research paper, my co-authors Atul and Alex share more detail on our assessment of the US equity market today, including how AI investment and pricing fit into the picture.
Current Conditions Provide Fertile Ground to Extend the AI-Led US Equity Rally
We are at the dawn of a significant capex surge. What we are seeing today is companies standing up initial infrastructure—a sort of “arms race” to build out basic AI capabilities. The technology is already an existential concern for some of the world’s most cash-rich companies (mostly in tech), and some of the largest pools of capital in the world are jockeying for exposure to the potential gains. This arms race means that we are likely to see continued investments in AI, which is also going to support profits for the likes of Nvidia and other companies that are at the epicenter of the infrastructure buildout. As discussed above, we expect new entrants will use AI techniques to disrupt established industries, likely prompting another wave of investment far beyond the tech industry.
Most of the stock rally thus far has narrowly benefited companies at the center of the initial AI infrastructure buildout, but not much is priced in for the beneficiaries of broader deployment. What’s more, the price increases in key infrastructure builders like Nvidia have been in line with changes in near-term earnings expectations, which have themselves been driven by announced capex plans, which are, again, nowhere close to the magnitude of capex we have witnessed in past technological transformations. And so far, neither economic nor financial investment flows have become unanchored from fundamentals, as they definitionally do in bubbles. Overall investor positioning in US equities is not light, but there is plenty of room for flows to pick up from here and turn frothy.
Cyclically, economic conditions are shaping up to be accommodative for a further equity rally. Now that inflation is back near target, the Fed is focused on the employment side of its dual mandate. That sets up a series of interest rate cuts into an economy where growth is doing OK and both household and corporate balance sheets are relatively healthy. Risk premiums are tight and global investors are up to their eyeballs in US equity risk, but history has shown that this type of macro environment can keep the music playing.
We think all this nets to an above-average chance of another leg up in equities tied to this technology—and we are continuously incorporating our thinking on this into our process at both macro and micro equity levels.
Below, we go into more detail on each of these points.
The High Stakes of Not Doing Enough Are Spurring an Investment Arms Race
Companies closest to the AI buildout are full steam ahead on capex, and we see no signs that will slow. As the comments below from recent earnings calls illustrate, many leaders of the largest companies closest to the technology are primarily worried about whether they are doing enough investment and see AI as a watershed moment.
- “The risk of underinvesting is dramatically greater than the risk of overinvesting.”—Sundar Pichai, Alphabet CEO, July 2024
- “I think that there’s a meaningful chance that a lot of the companies are overbuilding now and that you look back and you’re like, oh, we maybe all spent some number of billions of dollars more than we had to…Because the downside of being behind is that you’re out of position for, like, the most important technology for the next 10 to 15 years.”—Mark Zuckerberg, Meta cofounder and CEO, July 2024
- “The reality right now is that while we’re investing a significant amount in the AI space and in infrastructure, we would like to have more capacity than we already have today. I mean, we have a lot of demand right now, and I think it’s going to be a very, very large business for us.”—Andy Jassy, Amazon CEO, August 2024
These companies are following through on their statements with big—and increasing—capex plans. Mag 7 companies ex-Nvidia (which is actually on the receiving end of a lot of this capex spend) are expected to steadily increase capex to north of $250 billion annualized out to 2026, a huge sum (five times what they were spending in 2016). And since the end of 2022, analyst expectations for AI capex by those most directly involved in the initial AI buildout have steadily risen by almost $60 billion. These upward revisions show those companies (like Microsoft, Alphabet, Amazon, and Meta) and the analysts that cover them reckoning with the realization that faster investment in the AI buildout will be needed. Apple (which is relying on models developed externally by OpenAI and others) and Tesla have so far not seen similar upgrades to their high capex expectations.

Even with the currently announced plans, we are not yet seeing the scale of capex it has historically taken to support widespread technological adoptions and infrastructure buildouts. Below, we show the case of the 1990s, when corporates built out much of their computing infrastructure. What we have witnessed thus far is miniscule compared to the amount of spending that may be needed to deliver this technology. As noted above, over the next few years, we expect that new entrants will use ML techniques to disrupt established industries, likely prompting another wave of investment far beyond the tech industry.
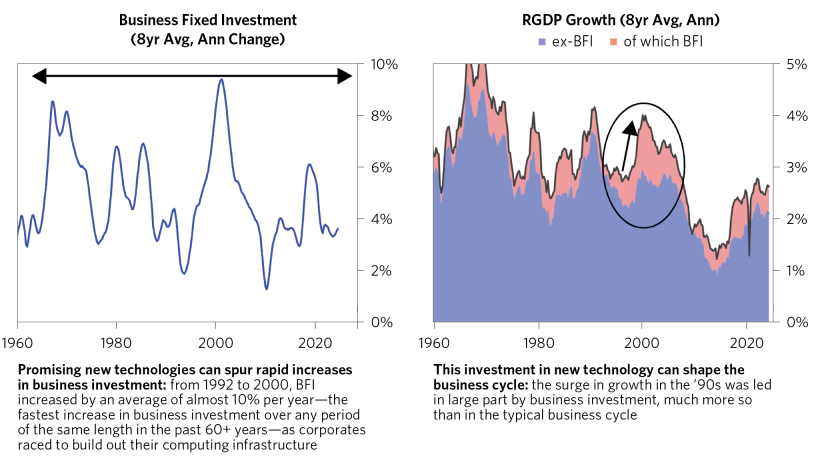
Markets Aren’t Yet Pricing in the Widespread Potential of AI
We don’t yet see much priced in for AI impact beyond the narrow set of stocks at the center of the AI infrastructure build. This point is represented simplistically below in a chart showing the returns of our AI slice since the release of ChatGPT in November 2022, which in many respects marked the introduction of generative AI to a broad audience and the start of increased investor enthusiasm around AI. The narrowness of the rally we’ve seen since then is not all that surprising, given there is so much unknown around how this plays out and who the winners and losers will be. That said, once markets start believing and extrapolating the broader impact of this technology, the pricing will likely broaden too.

The next three charts show how analysts’ bias-adjusted EPS estimates of Nvidia, other AI-exposed companies, and the rest of the S&P 500 have evolved since ChatGPT’s release. Nvidia, which is at the epicenter of the infrastructure boom, has seen increases in its expected earnings growth that are almost an order of magnitude greater than any other US company, and its actual EPS growth has accelerated dramatically, too. But as you move outward to companies further from the current AI buildout, the increases to expected EPS growth become more modest and largely just extrapolate forward changes in actual EPS growth.
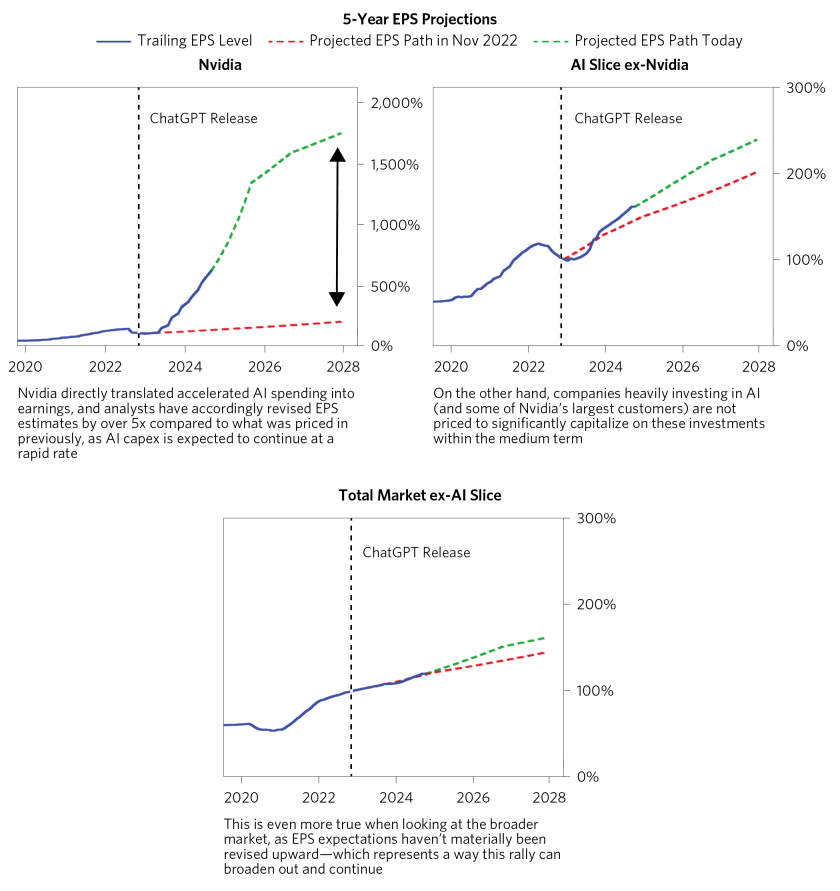
Macro Conditions Are Setting Up an Accommodative Policy Backdrop for Further AI Investment
Typically, the start of Fed rate-cut cycles coincides with the onset of a self-reinforcing economic slowdown, which puts cross-cutting pressures on equities: the cuts support valuations, but at the same time, cash flows and risk sentiment are usually deteriorating. By contrast, in this cycle the public sector has been the proactive player, kicking off an income-driven cycle with a notable lack of private sector credit excesses, contributing to economic resilience even in the face of aggressive Fed tightening. Now, with inflation moderating, the Fed is free to turn to easing while conditions are still OK and less likely to spiral downward. This sets up an unusual scenario where the Fed is set to ease proactively while cash flows and risk sentiment are still poised to support equities.
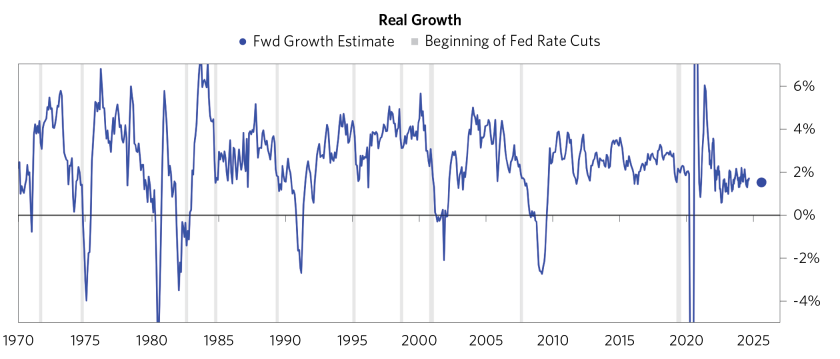
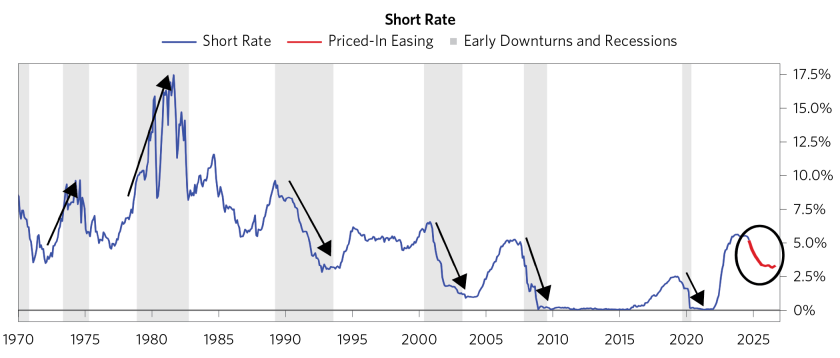
This easing into resilient growth is coming at a time when business balance sheets are quite healthy, with lots of cash on hand to spend and plenty of room to borrow more. They have plenty of resources to invest in AI, and a lower cost of capital is just one more reason to start ramping up.
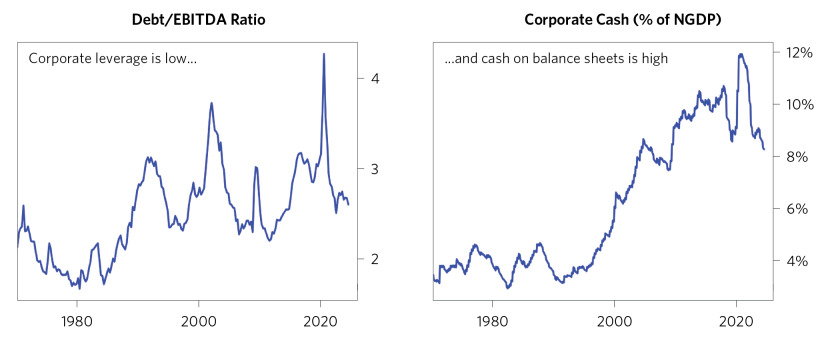
Historically, equities tend to do well when growth is durable, inflation isn’t a constraint, and policy remains easy enough that it does not short-circuit the expansion. Major technological breakthroughs can then drive returns especially high, such as with autos in the 1920s and the internet in the 1990s. The next table shows all “mid- to late-cycle” US rallies (defined here as periods with gains above 50% that were not coming out of a recession) since 1900 and the average growth, inflation, and policy conditions. The fact that these were mid-cycle rallies is notable; if these numbers were calculated trough-to-peak, they’d be even higher.

In aggregate, US equity risk premiums are tight and globally investors are heavily allocated to US equities, but history has shown this type of macro environment can push markets well beyond current levels. The current level of risk premiums is similar to what we saw for most of the 1960s and 1990s—and nothing like the compression in the run-up to the 1920s and 1990s bubbles—indicating that there’s room for risk premiums to get much more squeezed from here. As indicated below, pricing is stretched but by no means at historical extremes, with a potentially transformative technology on the horizon.

This research paper is prepared by and is the property of Bridgewater Associates, LP and is circulated for informational and educational purposes only. There is no consideration given to the specific investment needs, objectives, or tolerances of any of the recipients. Additionally, Bridgewater's actual investment positions may, and often will, vary from its conclusions discussed herein based on any number of factors, such as client investment restrictions, portfolio rebalancing and transactions costs, among others. Recipients should consult their own advisors, including tax advisors, before making any investment decision. This material is for informational and educational purposes only and is not an offer to sell or the solicitation of an offer to buy the securities or other instruments mentioned. Any such offering will be made pursuant to a definitive offering memorandum. This material does not constitute a personal recommendation or take into account the particular investment objectives, financial situations, or needs of individual investors which are necessary considerations before making any investment decision. Investors should consider whether any advice or recommendation in this research is suitable for their particular circumstances and, where appropriate, seek professional advice, including legal, tax, accounting, investment, or other advice. No discussion with respect to specific companies should be considered a recommendation to purchase or sell any particular investment. The companies discussed should not be taken to represent holdings in any Bridgewater strategy. It should not be assumed that any of the companies discussed were or will be profitable, or that recommendations made in the future will be profitable.
The information provided herein is not intended to provide a sufficient basis on which to make an investment decision and investment decisions should not be based on simulated, hypothetical, or illustrative information that have inherent limitations. Unlike an actual performance record simulated or hypothetical results do not represent actual trading or the actual costs of management and may have under or overcompensated for the impact of certain market risk factors. Bridgewater makes no representation that any account will or is likely to achieve returns similar to those shown. The price and value of the investments referred to in this research and the income therefrom may fluctuate. Every investment involves risk and in volatile or uncertain market conditions, significant variations in the value or return on that investment may occur. Investments in hedge funds are complex, speculative and carry a high degree of risk, including the risk of a complete loss of an investor’s entire investment. Past performance is not a guide to future performance, future returns are not guaranteed, and a complete loss of original capital may occur. Certain transactions, including those involving leverage, futures, options, and other derivatives, give rise to substantial risk and are not suitable for all investors. Fluctuations in exchange rates could have material adverse effects on the value or price of, or income derived from, certain investments.
Bridgewater research utilizes data and information from public, private, and internal sources, including data from actual Bridgewater trades. Sources include BCA, Bloomberg Finance L.P., Bond Radar, Candeal, CBRE, Inc., CEIC Data Company Ltd., China Bull Research, Clarus Financial Technology, CLS Processing Solutions, Conference Board of Canada, Consensus Economics Inc., DataYes Inc, DTCC Data Repository, Ecoanalitica, Empirical Research Partners, Entis (Axioma Qontigo Simcorp), EPFR Global, Eurasia Group, Evercore ISI, FactSet Research Systems, Fastmarkets Global Limited, The Financial Times Limited, FINRA, GaveKal Research Ltd., Global Financial Data, GlobalSource Partners, Harvard Business Review, Haver Analytics, Inc., Institutional Shareholder Services (ISS), The Investment Funds Institute of Canada, ICE Derived Data (UK), Investment Company Institute, International Institute of Finance, JP Morgan, JSTA Advisors, LSEG Data and Analytics, MarketAxess, Medley Global Advisors (Energy Aspects Corp), Metals Focus Ltd, MSCI, Inc., National Bureau of Economic Research, Neudata, Organisation for Economic Cooperation and Development, Pensions & Investments Research Center, Pitchbook, Rhodium Group, RP Data, Rubinson Research, Rystad Energy, S&P Global Market Intelligence, Scientific Infra/EDHEC, Sentix GmbH, Shanghai Metals Market, Shanghai Wind Information, Smart Insider Ltd., Sustainalytics, Swaps Monitor, Tradeweb, United Nations, US Department of Commerce, Verisk Maplecroft, Visible Alpha, Wells Bay, Wind Financial Information LLC, With Intelligence, Wood Mackenzie Limited, World Bureau of Metal Statistics, World Economic Forum, and YieldBook. While we consider information from external sources to be reliable, we do not assume responsibility for its accuracy.
This information is not directed at or intended for distribution to or use by any person or entity located in any jurisdiction where such distribution, publication, availability, or use would be contrary to applicable law or regulation, or which would subject Bridgewater to any registration or licensing requirements within such jurisdiction. No part of this material may be (i) copied, photocopied, or duplicated in any form by any means or (ii) redistributed without the prior written consent of Bridgewater® Associates, LP.
The views expressed herein are solely those of Bridgewater as of the date of this report and are subject to change without notice. Bridgewater may have a significant financial interest in one or more of the positions and/or securities or derivatives discussed. Those responsible for preparing this report receive compensation based upon various factors, including, among other things, the quality of their work and firm revenues.