The impacts of AI on the economy will depend both on how the technology evolves and how quickly and effectively it is implemented. We share how we are interpreting the growing literature on the topic and tracking the process in real time.
Editor’s Note: At Bridgewater, we are learning about the likely impact of AI in two ways. In this report, we share some of our research department’s thinking on the macroeconomic effects of AI. At the same time, we are also learning-by-doing in our AI lab, where we are bringing investors and AI experts together to explore cutting-edge use cases in an effort to generate alpha.
As investors, knowing what we don’t know is often more important than what we do know. We know that AI will matter for economies and markets in the coming years: in the past we have contextualized the possible impacts relative to the processes of globalization and industrial automation in the 1990s and 2000s, two slow-moving structural shifts that kept inflation low, led to rising inequality, supported corporate profits, and had meaningful political and social consequences. But how and when AI will matter for the economy remains highly uncertain. In this report, we bring you into our latest thinking on the likely economic impacts of AI, recognizing that at this stage there’s much more that we don’t know than do.
- What is happening so far? We are seeing rapid AI adoption in a few pockets of the economy, like customer contact centers and software engineering, where it is particularly compelling as a productivity enhancer that can reduce labor costs. The data is early and imperfect, but employment demand in these sectors looks to be slowing. However, these pressures are small in the context of the overall economy, where labor markets are tight. So far, the economic impacts of AI use look analogous to other recent technological developments that have mattered a great deal in certain industries but had only modest effects on aggregate growth and inflation.
- What do we know and not know about what is likely to happen next? We expect that AI will become a meaningful productivity enhancer across many more workflows, but this process is still in its infancy. So far, we see businesses experimenting with AI across a wide range of tasks, but few areas where automation has picked up a lot of steam. The main questions concern how widely AI will be adopted and how rapid and effective that process of adoption will be. There is a growing literature arguing that a very large share of the economy will integrate AI, but the methods underlying such estimates don’t have a track record of predicting the subsequent effects of adoption with any precision. And anticipating the effects is particularly challenging because the technology itself is evolving rapidly and often unpredictably, and the greatest productivity impacts may come from capabilities that have not yet emerged.
- Most studies estimate the peak impact to be around a decade or more away, and our base case remains that inflationary secular dynamics will be more dominant in the next few years than the deflationary impacts of AI. Unlocking the potential productivity benefits of the technology will require both the reskilling of workforces and meaningful organizational and process innovation, which historically are very slow-moving. The most likely outcome is that the integration of AI proceeds more slowly than other pressures that are already driving corporate decision making (e.g., the need to build resilient supply chains). But the range of external estimates is extremely wide, and this examination highlights the importance of recognizing what we don’t know and being prepared for multiple possibilities.
What Is Happening Today: Tracking the Integration of AI in Real Time
First, we look at what we know about how AI is being integrated into the economy today. Large language model (LLM) technology is new and evolving, so usage is changing quickly. And because the effects today are greatest in small subsets of the economy or specific workstreams, it is challenging to tease them out from aggregate growth statistics. While AI usage is likely to broaden from here, so far, the impacts are concentrated in a few industries, with only minor effects on aggregate growth and inflation.
LLMs are particularly well-suited for integration into workflows that are based on text in a constrained environment (i.e., where text or speech follow very consistent patterns). As such, the technology is being implemented very quickly in key functions that meet these criteria, and where its downsides (like its lack of precision or the still-murky regulatory landscape) are less acute—most notably, customer contact centers and software development. Below, we walk through what we’re seeing in these two sectors as case studies of the technology’s integration. For each, we outline the size of the sector, why it’s well-suited to integrating AI, and how that process is going.
Contact Centers
- It’s a small part of the economy. About 1.8% of the US workforce (~2.9 million people) are employed as customer service representatives, with ~17 million contact center employees globally.
- There is a compelling case to integrate AI. Customer contacts typically involve routine inquiries about basic information and are often text-based, making LLMs a good fit. And the desire to reduce labor costs is high, as labor makes up a very large share of total costs in the industry (Gartner estimates up to 95%).
- Adoption looks to be reasonably productivity-enhancing. Data is limited, and we’re still in the early stages. But one recent study of a company that implemented an AI tool to prompt technical chat support agents found an increase in issue resolution per hour by 14%, while a recent survey found that using AI tools to assist agents during contacts reduced average handle time by 27%. The most common use cases so far are assisting rather than replacing agents—for instance, by prompting agents or handling note-taking duties—but anecdotally, the range is wide; this podcast describes some workflows being fully replaced by AI.
- Adoption appears to be accelerating. Surveys are of varying quality, but all point to this trend. Already in 2021, before the technological breakthroughs often referred to as “generative AI,” one survey found that 71% of call centers were using AI tools, while another reported that 52% were in the midst of implementing AI. Since generative AI has come on the scene in a big way, one survey earlier this year found that 27% of contact centers were already using generative AI for customer-related activities and that another 47% planned to do so this year. Consistent with these company surveys, a majority of call center employees in a recent survey said that some of their work was currently being automated.
- We are likely seeing some impacts on employment. One recent study estimated that contact centers that are not using AI tools for customer experience would need to hire over twice as many new agents in 2023 compared to those that are. Identifying the employment impact in the official data is challenging, as we only see monthly data on employment in dedicated call center companies, which are a fraction of the total impacted universe (as this category does not include in-house customer support). This data shows a remarkably steep drop in employment (~10%) over the last 18 months as AI tools have been integrated into call center workstreams, which may be driven in part by AI integration (although other drivers, like labor shortages and rapidly rising domestic wages, may have also played a role). The only prior fall of a similar magnitude in recent decades was in 2002, when a handful of major call center companies conducted outsourcing operations. Meanwhile, wage growth has been strong despite job cuts, tracking very strong wage growth for lower-skill positions over the same period. This makes sense, as call centers compete with businesses like restaurants and hotels for labor, and reflects how deflationary impacts don’t reach the economy-wide level if they’re concentrated in small sectors. It’s also possible that remaining workers are more productive and hence more valuable with AI tools, as we saw with manufacturing automation.
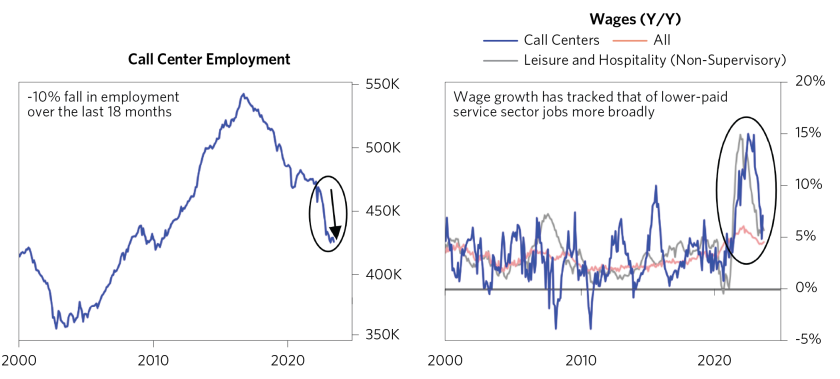
Software Development
- It’s small as a share of employment, but the potential impacts of automation are broader. Software developers make up only around 1.1% of US employment (~1.7 million people). But developers’ share of labor understates the impacts of enhancing their productivity, both because software developers produce more economic value than the average worker (mean hourly wages, a very rough proxy, are around twice the national average) and because the software and internet services sectors—where we estimate that software developers’ labor costs comprise roughly 15-20% of total costs—contribute an outsize share of profits among listed US companies (~10%).
- There is a compelling case to integrate AI. A scarcity of developers contributes to high labor costs; the median hourly wage for software developers is almost triple the national median wage.
- Adoption is meaningfully productivity-enhancing. In one controlled study, professional software developers granted access to GitHub Copilot completed an assigned task 56% faster than those who weren’t. Of developers who use Copilot, the tool writes 46% of their code on average (as of February 2023). The ultimate impact on productivity is more modest than these numbers indicate, as most surveys find that the typical software engineer spends only around half their time on coding and design tasks, but it is still large.
- Adoption appears to be accelerating. There aren’t good studies of the total usage of these tools, but anecdotally, it is widespread. GitHub says that 1.2 million people used Copilot in the year through June 2022, which is when they switched from a free to a paid model, but doesn’t provide good information on how frequently the tool was used, so we can’t know how much this statistic captures regular users as opposed to people just trying it out. Analogous tools from other companies (e.g., Replit’s Ghostwriter, Amazon’s CodeWhisperer) don’t have reliable usage statistics either. And any usage numbers for these purpose-built autocompletion tools would understate the extent to which AI is used to write code today, as the use of general-purpose LLMs like ChatGPT to write code is also widespread.
- We are seeing modest impacts on employment. It’s challenging to disaggregate the employment effects of AI tools from both broader employment trends in software engineering—like the partial unwinding of the massive COVID hiring spree earlier this year and the slowdown in certain “bubbly” tech—and increased demand for the subset of software engineers who can help develop AI technology. But there have been some employment impacts: the Challenger Report has linked just under 4,000 layoffs in tech to AI in the three months through July 2023 based on company statements (likely an understated measure), out of around 32,000 total layoffs that it recorded in the industry over the same period.
AI integration in these sectors is likely to continue, with contact centers having a clearer runway ahead. Gartner estimates that 10% of contact center interactions globally will be entirely automated with AI by 2026, up from 1.6% in mid-2022; McKinsey & Company estimates that in North America, where higher labor costs increase the incentive to automate, AI will further reduce the volume of human-serviced contacts by “up to 50%” as applications increasingly become labor-replacing.
The future impacts of AI integration in software development are less clear. Software engineering differs from jobs like customer service (or agriculture) because there is less of a natural limit on how much of it might be demanded at lower costs, increasing the potential growth impact of productivity enhancements. But we don’t yet know what people would do with cheaper software development, and the recent wave of layoffs in the industry suggests that the demand for software development might not be limitless in the short term. Meanwhile, the long-term employment impact is contingent on how the technology evolves from here: while the current undersupply of software engineers relative to everything they could accomplish means that improving their efficiency might not cause employment to fall much, this would change if we reach a point where AI tools fully match the capabilities of the typical software engineer. Finally, as we discuss further below, the biggest impacts on productivity will likely come from cheaper software engineering accelerating broader innovation, which will be a more gradual process.
So far, the integration of AI in these sectors doesn’t seem to be adding up to much in the total economy—it’s a modest offset to opposing inflationary pressures. The most important question is whether other industries will accelerate adoption, ramping up the impacts.
When we look more broadly, we see early experimentation with AI tools across a wide variety of tasks, but not many areas where the use of the technology is very widespread. In particular, many businesses are experimenting with ways to integrate the very new (and rapidly evolving) set of capabilities often referred to as “generative AI.” Our best guess is that generative AI tools will be a meaningful productivity enhancer across many workstreams, but this process is in its infancy; companies are still figuring out the technology, assessing the regulatory landscape, and preparing their workforces to use it. For example, a McKinsey & Company survey conducted in April 2023 across a global sample of companies (diverse across regions and industries) found that around one-third of respondents’ companies have started to use generative AI in at least one business function, but that companies were experimenting across a wide range of use cases, with no one function or use case yet seeing very widespread adoption.

What We Know and Don’t Know About What Is Likely to Happen Next
In the long run, economies grow either by having more people working or by having each person’s labor produce more—in other words, through either population or productivity growth. In major developed economies like the US, where population growth is low, productivity growth is the most important determinant of long-term economic growth. AI has the potential to accelerate productivity growth markedly from here. Below, we walk through when it might do so and by how much.
Most Studies Estimate Peak Impact Being Some Years Away, in Line with Past Technology Integrations
Most studies that take a view on the time frame of AI adoption forecast that the major productivity impacts will occur in the 2030s or 2040s, not the next few years. These estimates are largely informed by past productivity changes. Historically, general-purpose technologies that can be implemented widely across an economy—like electricity or computers—had a measurable impact on productivity around one to three decades after the technological capability emerged.
This meaningful lag between invention and macroeconomic impact happens for a few reasons. Most commercial uses of general-purpose technologies require complementary inventions, which are developed over time (e.g., the invention of the spreadsheet unlocked many of the commercial applications of the PC). Companies need to invest to implement new technologies and to retrain workers to use them effectively, and productivity may actually decrease as companies invest in a technology that isn’t yet productive. And, most crucially, the primary benefits of general-purpose technologies typically don’t come from doing the same processes faster, but rather from the totally new approaches that they enable—and it takes time to identify and adopt these approaches. For example, the electrification of American factories enhanced productivity in large part by making it possible to distribute machinery across factory floors instead of clustering machines around steam engines—but this development only happened many years after electrification, as factory owners at first simply swapped out steam engines for electric motors without rethinking their production lines.
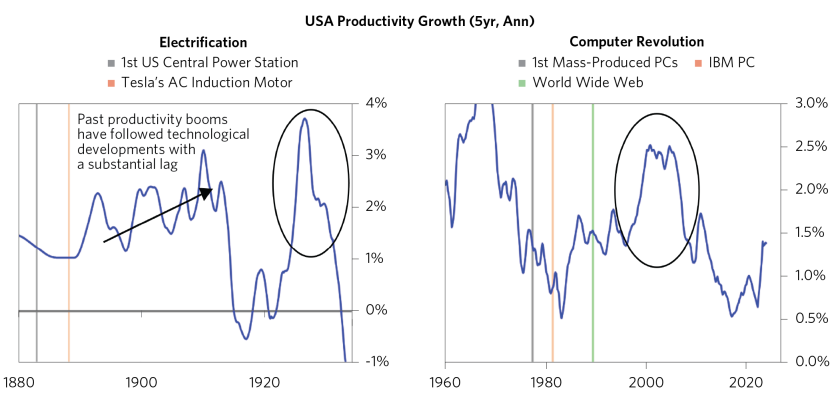
While history doesn’t always repeat, it’s worth noting that some estimates of AI adoption from a few years ago look naively optimistic today on how quickly businesses would start using the technology—for instance, this 2019 study from the MIT Technology Review, which concluded that 12% of all jobs in Asia would be automated by AI by 2024, or this 2017 study from PwC, which forecast that across most industries, something between 50-90% of potential AI use cases would already be implemented by 2023.
AI will flow through to productivity faster than past general-purpose technologies, but the peak impacts remain far off. AI will likely proceed more quickly for a few reasons. First, the uses of AI typically require less capex, so the marginal cost per unit of labor saved is lower than for past technologies. Second, that capex is more concentrated among major software players and therefore easier to coordinate; much of the adoption will take the form of AI tools being rolled out to software-as-a-service platforms that businesses already use. Finally, secularly low unemployment and high wage growth today may also increase the incentive to automate, although temporary cyclical pressures are unlikely to have a major impact on this decades-long process.
However, it’s not clear we should expect organizational innovation or retraining workers to happen faster than past waves of technological innovation—and the literature on past cases suggests that organizational innovation is the most important gate to unlocking the big productivity benefits of general-purpose technologies. In other words, the invention of the technology still needs to be followed by the “invention” of how to use it effectively, and this is typically a slow-moving process. Looking across this literature, our base case is that the integration of AI tools will proceed more slowly than the secular inflationary pressures that are already driving corporate decision making (e.g., the need to build resilient supply chains). But the range of external estimates is extremely wide, and it’s important to recognize what we don’t know and to be prepared for multiple possibilities.
There Is Tremendous Uncertainty Around How Large the Impacts of AI Will Be
Understanding when AI might impact productivity is only half the battle; it’s also important to assess what its effects might look like. There is a growing literature arguing that a very large share of the economy is likely to see AI integration. In the past, we’ve discussed the wide range of occupations that this literature identifies as vulnerable to automation, putting these potential impacts in the context of other structural changes in the labor force in recent decades. While the precise methodology varies across studies, recent studies from Goldman Sachs, McKinsey & Company, and OpenAI (among others) have adopted a “bottom-up” approach—using either the existing capabilities of AI technology or expert forecasts of how it will evolve alongside databases of the task content of jobs (like the O*NET database in the US or ESCO in Europe) to map the capabilities of AI to individual tasks that workers perform. From there, these studies scale up to sector and economy-wide estimates of the employment or productivity impacts of the technology.
These theoretical studies yield a very wide range of potential outcomes; at this stage, there’s much more that we don’t know than do about the effects of the technology. For instance, McKinsey & Company anticipates a 0.2-3.3 percentage point annual boost to productivity growth from automation through 2040, with 0.1-0.6 percentage points of that coming from generative AI, while Goldman Sachs forecasts a 0.3-2.9 percentage point increase in annual productivity growth from AI during its adoption period (for context, annual productivity growth is ~1.5% today). These ranges are massive—the difference between a productivity revolution and something more akin to what we’ve seen from other recent industrial technologies, which have mattered a lot for some industries but had only modest impacts on aggregate growth and inflation.

The “Task-Based” Approach Underlying the Research Is Inherently Uncertain
The methodology underlying these studies is very imprecise. Broad definitions of tasks only loosely correlate to what jobs entail, and AI’s ability to perform a certain type of task doesn’t mean that it can do it at the level or in the way that jobs require, making these estimates extremely sensitive to assumptions. Moreover, many seemingly “automatable” tasks are performed in work contexts where other skills or tasks not explicitly identified in a database are tacitly required for competent performance. And tasks entailed by a job often overlap, such that completing a task that AI is capable of might be a prerequisite for competent performance of another task that cannot be automated.
To illustrate the challenges of this type of exercise, below we show examples of “Detailed Work Activities” in the O*NET database, which is the most granular form of task data that is used in studies on AI’s potential impact (many use less granular task descriptions). For each of the activities shown below, we are confident that AI tools could do a meaningful amount of the work involved, but we could not tell you whether they could do 20% of all such work in the economy or 80%—and we don’t think others can either.

Most notably, such susceptibility estimates don’t have a track record of predicting subsequent effects of adoption with any precision. One study applied a similar task-matching method based on the O*NET database to past cases of technological innovation and found markedly different employment and wage impacts for the same degree of “exposure” in different cases. In robotics, an occupation moving from the 25th to 75th percentile of exposure to the technology was associated with declines in within-industry employment share of 9-18% and wage declines of 8-14%; the same move for software was about half as impactful, associated with 7-11% declines in within-industry employment share and 2-6% wage declines.
We Could Also Start Seeing Productivity Impacts Through an Acceleration of R&D, Rather Than the Automation of Existing Workflows
Perspectives that focus only on the share of existing tasks that AI could do are incomplete, as they neglect both how changes in the relative costs of different types of work (like software engineering) will change consumption patterns and, crucially, the effects of all the future technologies that AI could help to invent. Economists Erik Brynjolfsson, Anton Korinek, and Martin Neil Baily illustrate how much of the impact of AI could come from accelerating the highest value research and development work, separating the potential productivity boost from AI into two channels, which we illustrate below.
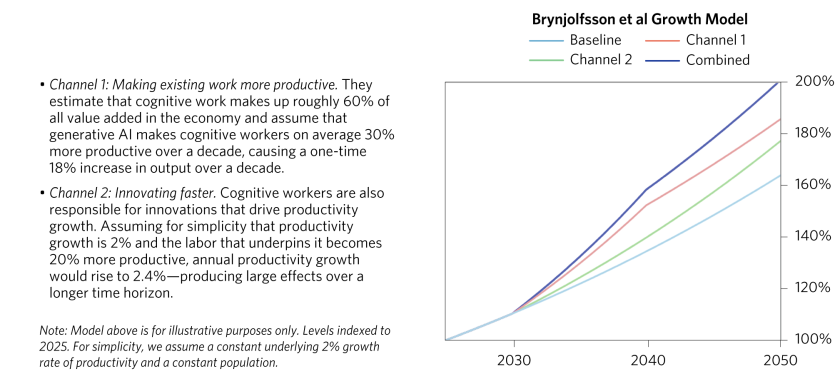
We could therefore see a much broader boost to productivity than that implied by the automation of existing workflows. But while the argument that AI will accelerate innovation is intuitively compelling, given AI’s extensive use cases in the research and startup ecosystems, it is far from clear that the bottleneck in enhancing productivity growth is the raw R&D; this article explores the idea that the bottleneck could be found in later essential steps like testing, getting ideas approved, manufacturing, and distribution.
Notably, research on past productivity waves has also concluded that many of the gains from new technologies flow through to consumer surplus that isn’t reflected in productivity statistics (or, sometimes, corporate earnings); AI may similarly lead to meaningful quality-of-life improvements that are missed in both official statistics and corporate cash flows. This point is most frequently discussed regarding the internet and other digital technologies, as much of the internet is free to use and digital technologies are so different in kind from past products that reported statistics could not be adjusted effectively for quality improvements. But it also applies to past technologies like automobiles, electric lighting, or medicines, where the benefits have not been captured well by official statistics. Below, we show the productivity growth data around computers and the internet—there was some boost, but not a massive one, and productivity growth reverted to around the 1970s-80s pace after the late 1990s and early 2000s.

Because the technology is still evolving, there are many more unknowns. The works referenced above are based either on today’s rough level of AI capabilities or current forecasts for how the technology will progress—but, of course, the great unknown is how the capabilities of the technology will evolve. The potential upside for growth and productivity depends on whether we see technological breakthroughs, which are tremendously challenging to predict; AI researchers have not anticipated many of the “emergent abilities” that models have gained as they have scaled up. At the most extreme, we may see the development of something like “artificial general intelligence” (AGI), a technology that could perform all the intellectual tasks that humans can. Such a technology would be truly transformative, as it could automate scientific advancement (among other contributions). Because an innovating AI could also produce ideas for making more and better such AI systems, we could get a very rapid feedback loop that produces explosive progress (although the impact of such a technology on growth might still be limited by physical or regulatory bottlenecks). But thoughtful estimates that we’ve seen of the probability of developing such a technology in the next two or three decades range widely, from close to 0% to around 50%, reflecting a deep uncertainty about the likely magnitude and speed of the technological breakthroughs ahead.

This research paper is prepared by and is the property of Bridgewater Associates, LP and is circulated for informational and educational purposes only. There is no consideration given to the specific investment needs, objectives, or tolerances of any of the recipients. Additionally, Bridgewater's actual investment positions may, and often will, vary from its conclusions discussed herein based on any number of factors, such as client investment restrictions, portfolio rebalancing and transactions costs, among others. Recipients should consult their own advisors, including tax advisors, before making any investment decision. This material is for informational and educational purposes only and is not an offer to sell or the solicitation of an offer to buy the securities or other instruments mentioned. Any such offering will be made pursuant to a definitive offering memorandum. This material does not constitute a personal recommendation or take into account the particular investment objectives, financial situations, or needs of individual investors which are necessary considerations before making any investment decision. Investors should consider whether any advice or recommendation in this research is suitable for their particular circumstances and, where appropriate, seek professional advice, including legal, tax, accounting, investment, or other advice.
The information provided herein is not intended to provide a sufficient basis on which to make an investment decision and investment decisions should not be based on simulated, hypothetical, or illustrative information that have inherent limitations. Unlike an actual performance record simulated or hypothetical results do not represent actual trading or the actual costs of management and may have under or overcompensated for the impact of certain market risk factors. Bridgewater makes no representation that any account will or is likely to achieve returns similar to those shown. The price and value of the investments referred to in this research and the income therefrom may fluctuate. Every investment involves risk and in volatile or uncertain market conditions, significant variations in the value or return on that investment may occur. Investments in hedge funds are complex, speculative and carry a high degree of risk, including the risk of a complete loss of an investor’s entire investment. Past performance is not a guide to future performance, future returns are not guaranteed, and a complete loss of original capital may occur. Certain transactions, including those involving leverage, futures, options, and other derivatives, give rise to substantial risk and are not suitable for all investors. Fluctuations in exchange rates could have material adverse effects on the value or price of, or income derived from, certain investments.
Bridgewater research utilizes data and information from public, private, and internal sources, including data from actual Bridgewater trades. Sources include BCA, Bloomberg Finance L.P., Bond Radar, Candeal, Calderwood, CBRE, Inc., CEIC Data Company Ltd., Clarus Financial Technology, Conference Board of Canada, Consensus Economics Inc., Corelogic, Inc., Cornerstone Macro, Dealogic, DTCC Data Repository, Ecoanalitica, Empirical Research Partners, Entis (Axioma Qontigo), EPFR Global, ESG Book, Eurasia Group, Evercore ISI, FactSet Research Systems, The Financial Times Limited, FINRA, GaveKal Research Ltd., Global Financial Data, Inc., Harvard Business Review, Haver Analytics, Inc., Institutional Shareholder Services (ISS), The Investment Funds Institute of Canada, ICE Data, ICE Derived Data (UK), Investment Company Institute, International Institute of Finance, JP Morgan, JSTA Advisors, MarketAxess, Medley Global Advisors, Metals Focus Ltd, Moody’s ESG Solutions, MSCI, Inc., National Bureau of Economic Research, Organisation for Economic Cooperation and Development, Pensions & Investments Research Center, Refinitiv, Rhodium Group, RP Data, Rubinson Research, Rystad Energy, S&P Global Market Intelligence, Sentix Gmbh, Shanghai Wind Information, Sustainalytics, Swaps Monitor, Totem Macro, Tradeweb, United Nations, US Department of Commerce, Verisk Maplecroft, Visible Alpha, Wells Bay, Wind Financial Information LLC, Wood Mackenzie Limited, World Bureau of Metal Statistics, World Economic Forum, YieldBook. While we consider information from external sources to be reliable, we do not assume responsibility for its accuracy.
This information is not directed at or intended for distribution to or use by any person or entity located in any jurisdiction where such distribution, publication, availability, or use would be contrary to applicable law or regulation, or which would subject Bridgewater to any registration or licensing requirements within such jurisdiction. No part of this material may be (i) copied, photocopied, or duplicated in any form by any means or (ii) redistributed without the prior written consent of Bridgewater® Associates, LP.
The views expressed herein are solely those of Bridgewater as of the date of this report and are subject to change without notice. Bridgewater may have a significant financial interest in one or more of the positions and/or securities or derivatives discussed. Those responsible for preparing this report receive compensation based upon various factors, including, among other things, the quality of their work and firm revenues.